Data science is a “concept to unify statistics, data analysis and their related methods” to “understand and analyse actual phenomena” with data. Data Science Training employs techniques and theories drawn from many fields within the broad areas of mathematics, statistics, information science, and computer science from the sub-domains of machine learning, classification, cluster analysis, data mining, databases, and visualization. The Data Science Certification Course enables you to gain knowledge of the entire life cycle of Data Science, analyse and visualise different data sets, different Machine Learning Algorithms like K-Means Clustering, Decision Trees, Random Forest, and Naive Bayes.
Data Science Certification Course using R
- Description
- Curriculum
- FAQ
- Reviews
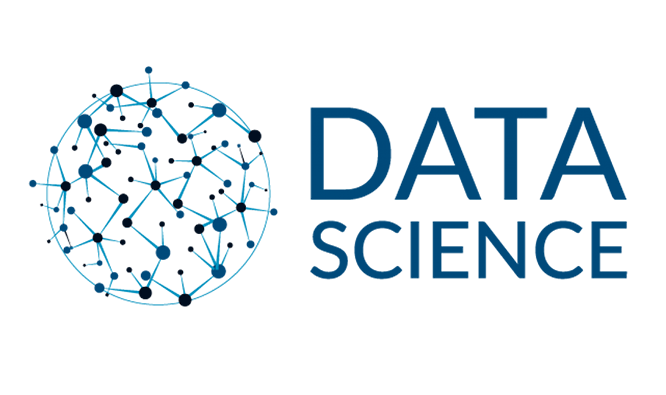
- In-depth knowledge of Data Science Life Cycle and Machine Learning Algorithms
- Comprehensive knowledge of various tools and techniques for Data Transformation
- The capability to perform Text Mining and Sentimental analyses on text data and gain an insight into Data Visualization and Optimization techniques
- The exposure to many real-life industry-based projects which will be executed in RStudio
- Projects which are diverse in nature covering media, healthcare, social media, aviation and HR
- Rigorous involvement of an SME throughout the Data Science Training to learn industry standards and best practices
- Gain insight into the ‘Roles’ played by a Data Scientist
- Analyze several types of data using R
- Describe the Data Science Life Cycle
- Work with different data formats like XML, CSV, etc.
- Learn tools and techniques for Data Transformation
- Discuss Data Mining techniques and their implementation
- Analyze data using Machine Learning algorithms in R
- Explain Time Series and it’s related concepts
- Perform Text Mining and Sentimental analyses on text data
- Gain insight into Data Visualization and Optimization techniques
- Understand the concepts of Deep Learning
- Developers aspiring to be a ‘Data Scientist’
- Analytics Managers who are leading a team of analysts
- Business Analysts who want to understand Machine Learning (ML) Techniques
- Information Architects who want to gain expertise in Predictive Analytics
- ‘R’ professionals who wish to work Big Data
- Analysts wanting to understand Data Science methodologies
There is no specific pre-requisite for Data Science Training. However, a basic understanding of R can be beneficial. Certs Learning offers you a complimentary self-paced course, i.e. “R Essentials” when you enroll in Data Science Training.
-
1Introduction to Data Science
Goal: Get an introduction to Data Science in this Module and see how Data Science helps to analyze large and unstructured data with different tools.
Objectives: At the end of this Module, you should be able to:
- Define Data Science
- Discuss the era of Data Science
- Describe the Role of a Data Scientist
- Illustrate the Life cycle of Data Science
- List the Tools used in Data Science
- State what role Big Data and Hadoop, Python, R and Machine Learning play in Data Science
Topics:
- What is Data Science?
- What does Data Science involve?
- Era of Data Science
- Business Intelligence vs Data Science
- Life cycle of Data Science
- Tools of Data Science
- Introduction to Python
-
2Statistical Inference
Learning Objectives - In this module, you will learn about different statistical techniques and terminologies used in data analysis.
Topics:
- What is Statistical Inference?
- Terminologies of Statistics
- Measures of Centers
- Measures of Spread
- Probability
- Normal Distribution
- Binary Distribution
-
3Data Extraction, Wrangling and Exploration
Learning Objectives - Discuss the different sources available to extract data, arrange the data in structured form, analyze the data, and represent the data in a graphical format.
Topics:
- Data Analysis Pipeline
- What is Data Extraction
- Types of Data
- Raw and Processed Data
- Data Wrangling
- Exploratory Data Analysis
- Visualization of Data
Hands-On/Demo:
- Loading different types of dataset in R
- Arranging the data
- Plotting the graphs
-
4Introduction to Machine Learning
Learning Objectives - Get an introduction to Machine Learning as part of this module. You will discuss the various categories of Machine Learning and implement Supervised Learning Algorithms.
Topics:
- What is Machine Learning?
- Machine Learning Use-Cases
- Machine Learning Process Flow
- Machine Learning Categories
- Supervised Learning algorithm: Linear Regression and Logistic Regression
Hands-On/Demo:
- Implementing Linear Regression model in R
- Implementing Logistic Regression model in R
-
5Classification Techniques
Learning Objectives - In this module, you should learn the Supervised Learning Techniques and the implementation of various techniques, such as Decision Trees, Random Forest Classifier, etc.
Topics:
- What are classification and its use cases?
- What is Decision Tree?
- Algorithm for Decision Tree Induction
- Creating a Perfect Decision Tree
- Confusion Matrix
- What is Random Forest?
- What is Naive Bayes?
- Support Vector Machine: Classification
Hands-On/Demo:
- Implementing Decision Tree model in R
- Implementing Linear Random Forest in R
- Implementing Naive Bayes model in R
- Implementing Support Vector Machine in R
-
6Unsupervised Learning
Learning Objectives - Learn about Unsupervised Learning and the various types of clustering that can be used to analyze the data.
Topics:
- What is Clustering & its use cases
- What is K-means Clustering?
- What is C-means Clustering?
- What is Canopy Clustering?
- What is Hierarchical Clustering?
Hands-On/Demo:
- Implementing K-means Clustering in R
- Implementing C-means Clustering in R
- Implementing Hierarchical Clustering in R
-
7Recommender Engines
Learning Objectives - In this module, you should learn about association rules and different types of Recommender Engines.
Topics:
- What is Association Rules & its use cases?
- What is Recommendation Engine & it’s working?
- Types of Recommendations
- User-Based Recommendation
- Item-Based Recommendation
- Difference: User-Based and Item-Based Recommendation
- Recommendation use cases
Hands-On/Demo:
- Implementing Association Rules in R
- Building a Recommendation Engine in R
-
8Text Mining
Learning Objectives - Discuss Unsupervised Machine Learning Techniques and the implementation of different algorithms, for example, TF-IDF and Cosine Similarity in this Module.
Topics:
- The concepts of text-mining
- Use cases
- Text Mining Algorithms
- Quantifying text
- TF-IDF
- Beyond TF-IDF
Hands-On/Demo:
- Implementing Bag of Words approach in R
- Implementing Sentiment Analysis on Twitter Data using R
-
9Time Series
Learning Objectives - In this module, you should learn about Time Series data, different component of Time Series data, Time Series modeling - Exponential Smoothing models and ARIMA model for Time Series Forecasting.
Topics:
- What is Time Series data?
- Time Series variables
- Different components of Time Series data
- Visualize the data to identify Time Series Components
- Implement ARIMA model for forecasting
- Exponential smoothing models
- Identifying different time series scenario based on which different Exponential Smoothing model can be applied
- Implement respective ETS model for forecasting
Hands-On/Demo:
- Visualizing and formatting Time Series data
- Plotting decomposed Time Series data plot
- Applying ARIMA and ETS model for Time Series Forecasting
- Forecasting for given Time period
-
10Deep Learning
View the recorded session of the class available in your LMS.
You can attend the missed session, in any other live batch.