Data Analyst Masters Program
- Description
- Curriculum
- Reviews
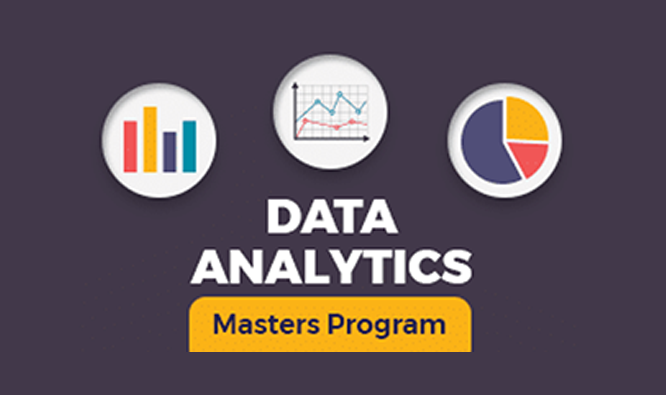
Data Analytics Masters Program makes you proficient in tools and systems used by Data Analytics Professionals. It includes in-depth training on Statistics, Data Analytics with R, SAS and Tableau. The curriculum has been determined by extensive research on 5000+ job descriptions across the globe.
-
1Statistics Essentials for Analytics
Understanding the Data
Objectives: At the end of this Module, you should be able to:
- Understand various data types
- Learn Various variable types
- List the uses of variable types
- Explain Population and Sample
- Discuss sampling techniques
- Understand Data representation
Topics:
- Introduction to Data Types
- Numerical parameters to represent data
- a. Mean
- b. Mode
- c. Median
- d. Sensitivity
- e. Information Gain
- f. Entropy
- Statistical parameters to represent data
Probability and its uses
Objectives: At the end of this Module, you should be able to:
- Understand rules of probability
- Learn about dependent and independent events
- Implement conditional, marginal and joint probability using Bayes Theorem
- Discuss probability distribution
- Explain Central Limit Theorem
Topics:
- Uses of probability
- Need of probability
- Bayesian Inference
- Density Concepts
- Normal Distribution Curve
Statistical Inference
Objectives: At the end of this Module, you should be able to:
- Understand concept of point estimation using confidence margin
- Draw meaningful inferences using margin of error
- Explore hypothesis testing and its different levels
Topics:
- Point Estimation
- Confidence Margin
- Hypothesis Testing
- Levels of Hypothesis Testing
Data Clustering
Objectives: At the end of this module, you should be able to:
- Understand concept of association and dependence
- Explain causation and correlation
- Learn the concept of covariance
- Discuss Simpson’s paradox
- Illustrate Clustering Techniques
Topics:
- Association and Dependence
- Causation and Correlation
- Covariance
- Simpson’s Paradox
- Clustering Techniques
Testing the Data
Objectives: At the end of this module, you should be able to:
- Understand Parametric and Non-parametric Testing
- Learn various types of parametric testing
- Discuss experimental designing
- Explain a/b testing
Topics:
- Parametric Test
- Parametric Test Types
- Non- Parametric Test
- Experimental Designing
- A/B testing
Regression Modelling
Objectives: At the end of this module, you should be able to:
- Understand the concept of Linear Regression
- Explain Logistic Regression
- Implement WOE
- Differentiate between heteroscedasticity and homoscedasticity
- Learn concept of residual analysis
Topics:
- Logistic and Regression Techniques
- Problem of Collinearity
- WOE and IV
- Residual Analysis
- Heteroscedasticity
- Homoscedasticity
-
2Data Analytics with R Certification Training
Introduction to Data Analytics
Learning Objectives - This module introduces you to some of the important keywords in R like Business Intelligence, Business Analytics, Data and Information. You can also learn how R can play an important role in solving complex analytical problems. This module tells you what is R and how it is used by the giants like Google, Facebook, Bank of America, etc. Also, you will learn use of `R` in the industry, this module also helps you compare R with other software in analytics, install R and its packages.
Topics - Introduction to terms like Business Intelligence, Business Analytics, Data, Information, how information hierarchy can be improved/introduced, understanding Business Analytics and R, knowledge about the R language, its community and ecosystem, understand the use of `R` in the industry, compare R with other software in analytics, Install R and the packages useful for the course, perform basic operations in R using command line, learn the use of IDE R Studio and Various GUI, use the ‘R help’ feature in R, knowledge about the worldwide R community collaboration.
Introduction to R Programming
Learning Objectives - This module starts from the basics of R programming like datatypes and functions. In this module, we present a scenario and let you think about the options to resolve it, such as which datatype should one to store the variable or which R function that can help you in this scenario. You will also learn how to apply the `join` function in SQL.
Topics - The various kinds of data types in R and its appropriate uses, the built-in functions in R like: seq(), cbind (), rbind(), merge(), knowledge on the various subsetting methods, summarize data by using functions like: str(), class(), length(), nrow(), ncol(), use of functions like head(), tail(), for inspecting data, Indulge in a class activity to summarize data, dplyr package to perform SQL join in R
Data Manipulation in R
Learning Objectives - In this module, we start with a sample of a dirty data set and perform Data Cleaning on it, resulting in a data set, which is ready for any analysis. Thus using and exploring the popular functions required to clean data in R.
Topics - The various steps involved in Data Cleaning, functions used in Data Inspection, tackling the problems faced during Data Cleaning, uses of the functions like grepl(), grep(), sub(), Coerce the data, uses of the apply() functions.
Data Import Techniques in R
Learning Objectives - This module tells you about the versatility and robustness of R which can take-up data in a variety of formats, be it from a csv file to the data scraped from a website. This module teaches you various data importing techniques in R.
Topics - Import data from spreadsheets and text files into R, import data from other statistical formats like sas7bdat and spss, packages installation used for database import, connect to RDBMS from R using ODBC and basic SQL queries in R, basics of Web Scraping.
Exploratory Data Analysis
Learning Objectives - In this module, you will learn that exploratory data analysis is an important step in the analysis. EDA is for seeing what the data can tell us beyond the formal modeling or hypothesis. You will also learn about the various tasks involved in a typical EDA process.
Topics - Understanding the Exploratory Data Analysis(EDA), implementation of EDA on various datasets, Boxplots, whiskers of Boxplots. understanding the cor() in R, EDA functions like summarize(), llist(), multiple packages in R for data analysis, the Fancy plots like the Segment plot, HC plot in R.
Data Visualization in R
Learning Objectives - In this module, you will learn that visualization is the USP of R. You will learn the concepts of creating simple as well as complex visualizations in R.
Topics - Understanding on Data Visualization, graphical functions present in R, plot various graphs like tableplot, histogram, Boxplot, customizing Graphical Parameters to improvise plots, understanding GUIs like Deducer and R Commander, introduction to Spatial Analysis.
Data Mining: Clustering Techniques
Learning Objectives - This module lets you know about the various Machine Learning algorithms. The two Machine Learning types are Supervised Learning and Unsupervised Learning and the difference between the two types. We will also discuss the process involved in `K-means Clustering`, the various statistical measures you need to know to implement it in this module.
Topics - Introduction to Data Mining, Understanding Machine Learning, Supervised and Unsupervised Machine Learning Algorithms, K-means Clustering.
Data Mining: Association Rule Mining & Collaborative filtering
Learning Objectives - In this module, you will learn how to find the associations between many variables using the popular data mining technique called the "Association Rule Mining", and implement it to predict buyers` next purchase. You will also learn a new technique that can be used for recommendation purpose called "Collaborative Filtering". Various real-time based scenarios are shown using these techniques in this module.
Topics - Association Rule Mining, User Based Collaborative Filtering (UBCF), Item Based Collaborative Filtering (IBCF)
Linear and Logistic Regression
Learning Objectives - This module touches the base of `Regression Techniques’. Linear and logistic regression is explained from the basics with the examples and it is implemented in R using two case studies dedicated to each type of Regression discussed.
Topics - Linear Regression, Logistic Regression.
Anova and Sentiment Analysis
Learning Objectives - This module tells you about the Analysis of Variance (Anova) Technique. The algorithm and various aspects of Anova have been discussed in this module. Additionally, this module also deals with Sentiment Analysis and how we can fetch, extract and mine live data from Twitter to find out the sentiment of the tweets.
Topics - Anova, Sentiment Analysis.
Data Mining: Decision Trees and Random Forest
Learning Objectives - This module covers the concepts of Decision Trees and Random Forest. The algorithm for creation of trees and classification of decision trees and the various aspects like the Impurity function Gini Index, Pruning, Entropy etc are extensively taught in this module. The algorithm of Random Forests is discussed in a step-wise approach and explained with real-life examples. At the end of the class, these concepts are implemented on a real-life data set.
Topics - Decision Tree, the 3 elements for classification of a Decision Tree, Entropy, Gini Index, Pruning and Information Gain, bagging of Regression and Classification Trees, concepts of Random Forest, working of Random Forest, features of Random Forest, among others.
Project Work
Learning Objectives - This module discusses various concepts taught throughout the course and their implementation in a project.
Topics - Analyze census data to predict insights on the income of the people, based on the factors like: age, education, work-class, occupation using Decision Trees, Logistic Regression and Random Forest. Analyze the Sentiment of Twitter data, where the data to be analyzed is streamed live from twitter and sentiment analysis is performed on the same.
-
3SAS Training and Certification
Getting started with SAS
Goal: In this module, you will learn to use the SAS Environment and program flow in SAS with specifications of Data and Proc steps. Also, you will learn to install SAS University Edition on your system.
Objective:
At the end of this module, you should be able to:
• Install SAS University Edition
• Express SAS as a language
• Describe the rules for the definition of SAS names
• Define the different datatypes, datasets in SAS
• Explain the significance of Data/Procedural step
• Run a simple SAS program
• Exercise better control over Data Step Programming using PDV.
Topics:
• Use Cases of SAS implementation
• Installing SAS University Edition
• Explore SAS GUI, SAS window and its contents
• Rules for definition of a SAS name
• Different Datatypes in SAS
• Use of Formats and Informats in SAS
• Illustration of SAS Datasets
• The Data step and procedural step
• Creating an Instream SAS Dataset
• Basic Procedural statements
• Assessing Input Buffer and Program Data Vector (PDV)
Hands On:
• Navigating the SAS windows environment
• Nuts and bolts of SAS programming steps
• Converting data as per required format
• Creating a dataset using CARDS
• Generating the output using PROC PRINT
Processing and Integrating with the Data Step
Goal: This module introduces you to import datasets and control the import of datasets into SAS and ultimately concatenating them.
Objective: At the end of this module, you should be able to:
• Bring in your external data into SAS and modifying it.
• Describe and subset permanent datasets.
• Modify/Delete existing columns
• Concatenate Datasets
Topics:
• Import of Data in SAS
• Manipulating influx of datasets into SAS
• Creating a new variable
• Working with Temporary and Permanent Datasets
• Set and Merge Statements
Hands On:
• Demonstrating import of external data within SAS using INFILE
• Demonstrating import of external data within SAS using PROC IMPORT
• Creating Permanent Datasets
• Performing operations with KEEP, DROP and RENAME and LABEL options
• Constructing a new variable
• Creating integrated datasets using SET/MERGE
Customizing Datasets
Goal: This module introduces the concept of looping and its implementation in the SAS programming language. You will explore arrays and functions to simplify complications in SAS programming.
Objective: At the end of this module, you should be able to:
• Demonstrate dependent and independent looping
• Master data step programming with SAS functions
• Simplify processing with SAS Arrays
Topics:
• Conditional& Iterative Processing
• SAS Arrays
• Useful SAS Functions
PUT/INPUT Functions
Date/Time Functions
Numeric Functions
Character Functions
Hands On:
• Use of DO, DO WHILE, DO UNTIL, IF ELSE, ELSE, ELSE IF
• Demonstrate the use of arrays in SAS
• Operation with INPUT/PUT functions
• Writing a Program with date/time functions
• Performing programming with Numeric functions
• Executing program with Character Functions
Advanced SAS Procedures
Goal: You will learn the analysis of your statistical data with advanced SAS procedures
Objective: At the end of this module, you should be able to:
• Explain Statistical Procedures
• Define priorities to your statistical data
• Discuss statistics associated with a variable
• Express correlation between two or more variables
Topics:
• Proc Dataset
• Proc Format
• Proc Sort
• Proc Means
• Proc Freq
• Proc Surveyselect
• Proc Transpose
• Proc Summary
• Proc Rank
• Proc Corr
• Proc Univariate
Hands On:
• Obtaining statistical means of variables
• Checking the degree of dependence within different variables
• Generating ranks for statistical data
• Performing re-structuring of data
• Conducting sampling: Random and Stratified
Advanced Statistical Proficiency with SAS
Goal: You will learn to model estimate and classify events based on the values of dependent variables. You will be taught to perform different types of clustering methodologies to bunch your observations.
Objective: At the end of this module, you should be able to:
• Define clustering and its types
• Explain clustering algorithms
• Nest data in different clusters
• Analyse the regression between two or multiple variables
• Model and estimate an event based on Logits
Topics:
• Introduction to Clustering
• Hierarchical Clustering
• Non-Hierarchical Clustering (K means Clustering)
• Simple and Multiple Linear Regression
• Logistic Regression
Hands On:
• Demonstrate the use of PROC CLUSTER
• Writing a SAS advanced program with PROC FASTCLUS
• Performing operations on regression with PROC REG
• Demonstrate modelling using PROC LOGISTIC
Optimization of variables and SAS ODS
Goal: You will learn to reduce the degree of complexity of a problem by developing& resolving optimization models and ultimately routing detailed presentation output from SAS in desired formats and destination.
Objective: At the end of this module, you should be able to:
• Discuss data optimization
• Acquire optimized models for complex problems
• Resolve optimization models
• Describe the Output Delivery System in SAS
• Explain the use of ODS statement for creation of different file types
Topics:
• What is Data Optimization?
• Realizing Optimization Models
• Using Proc Optmodel
• Solving the Rosenbrock Problem
• Introduction to ODS
• Benefits of using ODS
• Generating rtf file
• Generating pdf file
• Generating html file
• Generating doc file
Hands On:
• Writing a program with PROC OPTMODEL
• Solving the optimization model using SOLVE
• Extracting optimized outputs
• Routing quality presentation files
• Create html files
• Create rtf file
• Create pdf and doc files
PROC SQL
Goal: You will learn to invoke SQL in SAS.
Objective: At the end of this module, you should be able to:
• Write SQL code using various styles of the SELECT statement
• Use CASE/WHEN clauses for conditionally processing the data
• Systemize appearance of observations
• Join data from two or more data sets
Topics:
• Creating new tables
• The SELECT statement
• Sorting Data
• The CASE expression
• Other SELECT statement clauses
• JOINS and UNIONS
Hands On:
• Demonstrating the SQL Procedure
• Writing the SELECT clause
• Application of WHERE clause
• Merging Datasets
• Using ORDER BY clause
Using SAS Macros
Goal: You will explore how to automate a complex repeated process in SAS with the use of Macros.
Objective: At the end of this module, you should be able to:
• Define Macro Concept
• Automate a task that you perform repeatedly
• Explain Macro Step
• Express Macro step as a combination of different variables
Topics:
• Introduction to Macros
• Benefits of using SAS Macros
• Macro Code Constituents
• Macro Variables
• Macro Step
• Positional Parameters to Macros
Hands On:
• Demonstrate the use of Macro variables in SAS
• Writing a Macro code to simplify a program
• Demonstrate Macro step programming
• Performing Macro coding by passing parameters
-
4Tableau Training & Certification
Introduction to Data Visualization
Goal : Give a brief idea of data visualization and introduce Tableau 10
Objectives:
- Identify the prerequisites, goal, objectives, methodology, material, and agenda for the course
- Discuss the basic of Data Visualization
- Get a brief idea about Tableau, establish connection with the dataset, perform Joins operation on the data set
Topics:
- Data Visualization
- Introducing Tableau 10.0
- Establishing Connection
- Joins and Union
- Data Blending
Hands On:
- Establishing connection with the files, Introducing important UI components (ShowMe, Fit Axes)
- Perform Cross Joins between the dataset
Visual Analytics
Goal : Learn to manage your dataset and analyze things visually with the help of Marks Card and “highlighting” feature.
Objectives:
- Manage extracts and metadata (by creating hierarchy and folders)
- Describe what is Visual Analytics, why to use it, and it’s various scopes
- Explain aggregating and disaggregating data and how to implement data granularity using marks card on aggregated data
- Describe what is highlighting, with the help of a use-case
- Illustrate basic graphs including bar graph, line graph, pie chart, dual axis graph, and area graph with dual axis
Topics:
- Managing Extracts
- Managing Metadata
- Visual Analytics
- Data Granularity using Marks Card
- Highlighting
- Introduction to basic graphs
Hands On:
- Creating Extracts, Hierarchy, Folders
- All the features of Marks Card Shelve with use case provided
- Power of Highlighting in the visualization using the Use-case
- How to create basic graphs in Tableau10.x
Visual Analytics in depth I
Goal : This module presents to you the granular content of Visual analytics, covering various techniques to perform sorting, filtering and grouping on the dataset.
Objectives:
- Perform sorting techniques including quicksort, using measures, using header and legend, and sorting using pill with the help of a use case.
- Master yourself into various filtering techniques such as Parametrized filtering, Quick Filter, Context Filter. Learn about various filtering option available with the help of use case and different scenarios.
- Illustrate grouping using data-window, visual grouping, and Calculated Grouping (Static and Dynamic).
- Illustrate some more graphical visualization including Heat Map, Circle Plot, Scatter Plot, and Tree Maps.
Topics:
- Sorting.
- Filtering.
- Grouping
- Graphical Visualization
Hands On:
- Quick Sort, Sorting using measure, Sorting using header and legends, sorting using pill(use-case).
- Filtering Use cases covering different options (General, Wildcard, Conditional).
- Interactive Filter, Quick Filter, Context Filter.
- Grouping using Data Window, Visual Grouping, Calculated Grouping (Static and Dynamic).
Visual Analytics in depth II
Goal : This module presents to you Visual analytics in a more granular manner thereby letting you to dive deep into the content. It covers various advanced techniques of analyzing data including, forecasting, trend lines, reference lines, clustering, parametrized concepts, and creating sets.
Objectives:
- Explain the basic concepts of sets followed by Creating sets using Marks Card, computation sets and combined sets
- Describe the concepts of forecasting with the help of Forecasting problem as a use-case
- Discuss the basic concept of clustering in Tableau
- Add Trend lines and reference line to your visualization
- Discuss about Parameter in depth using Sets and Filter
Topics:
- Sets
- Forecasting
- Clustering
- Trend Lines.
- Reference Lines.
- Parameters
Hands On:
- Create sets using marks card, Computation sets, and Combined sets
- Forecasting using Precise Range
- Methods of clustering
- Adding trend line and reference line (along with various options available for them)
- Parameter using sets and filter
Dashboard and Stories
Goal : Learn all about Dashboards and Stories in Tableau.
Objectives:
- Describe the basic concepts of Dashboard and its UI.
- Build a dashboard by adding sheets and object into it
- Modify the view and layout.
- Edit your dashboard, how it should appear on phones or tablets.
- Create an interactive dashboard using actions (filter, highlighting, URL).
- Create stories for your Visualization and Dashboards.
Topics:
- Introduction to Dashboard.
- Creating a Dashboard Layout.
- Designing Dashboard for Devices.
- Dashboard Interaction - Using Action.
- Introduction to Story Point.
Hands On:
- Creating Dashboard and learning its UI component.
- Changing the layout of the dashboard.
- Using Device Designer to create dashboard for devices.
- Create an interactive dashboard using actions (Filter, Highlight, URL).
- Creating story with dashboard.
Mapping
Goal: This module helps you in understanding mapping in detail, editing unrecognized and ambiguous location, and creating customized geocoding. Learn about polygon map and Web Mapping Service, and finally, add background images with self-generated coordinates.
Objectives:
- Map the coordinates on the map, plot geographic data, and use a layered view to get the street view of the area.
- Edit the ambiguous and unrecognized location plotted on the map.
- Customize territory on a polygon map.
- Connect to the WMS Server, use a WMS background map and saving it.
- Add a background image and generate its coordinate and plot the points.
Topics:
- Introduction to Maps.
- Editing Unrecognized Locations.
- Custom Geocoding.
- Polygon Maps.
- Web Mapping Services.
- Background Images.
Hands-On:
- Plot the coordinate points on the map, plotting the geographic data, Street View using the layered view.
- Editing Unrecognized and ambiguous location
- Custom Geocoding.
- Creating a custom territory, building a polygon map.
- Establishing a connection with the WMS Server a WMS background map and saving it.
- Adding a background image and generate coordinates and finally plotting points.
Calculation
Goal: This module will help you in creating basic calculations including string manipulation, basic arithmetic calculations, date math, logic statements and quick table calculations. Along with this, you will be also introduced to LOD expressions with the help of use cases.
Objectives:
- Perform Calculations using various types of functions such as Number, String, Date, Logical, and Aggregate.
- In addition, you will get to know about Quick Table Calculation.
- Cover the following LOD expressions – Fixed, Included, and Excluded.
Topics:
- Introduction to Calculation: Number Functions, String Functions, Date Functions, Logical Functions, Aggregate Functions.
- Introduction to Table Calculation.
- Introduction to LOD expression: Fixed LOD, Included LOD, Excluded LOD
Hands-On:
- All Functions (Number, String, Date, Logical, Aggregate)
- Table Calculation.
- LOD expressions.
LOD Problem Sets & Hands on
Goal: This module will explain the scenarios where you can implement LOD expressions. This is showcased with the help of a set of problems.
Objectives:
- Tackle complex scenarios by using LOD expressions.
Hands-On:
- Use Case I - Count Customer by Order.
- Use Case II - Profit per Business Day.
- Use Case III - Comparative Sales.
- Use Case IV - Profit Vs Target
- Use Case V - Finding the second order date.
- Use Case VI - Cohort Analysis
Charts
Goal : Plot various types of Charts using Tableau 10 and have extensive hands-on on industry use cases.
Topics :
- Box and Whisker`s Plots
- Gantt Charts
- Waterfall Charts
- Pareto Charts
- Control Charts
- Funnel Charts
Hands On:
- Extensive hands-on on the above topics
Integrating Tableau with R and Hadoop
Goal: This module introduces you to the concept of Big Data, Hadoop, and R. You discuss the integration between Tableau and R and finally publish your workbook on Tableau Server.
Objectives:
- You will know the basics of Big Data, Hadoop, and R.
- You will discuss the integration between Hadoop and R and will integrate R with Tableau.
- In addition, you will get to publish your workbook on Tableau Server.
Topics:
- Introduction to Big Data
- Introduction to Hadoop
- Introduction to R
- Integration among R and Hadoop
- Calculating measure using R
- Integrating Tableau with R
- Integrated Visualization using Tableau
Hands-On:
- Installing Rserve package in R
- Integrating Tableau and R
- Publishing your workbook on Tableau Server.